In this blog post, we introduce Vid2Avatar, an exciting new method that revolutionizes the creation of lifelike 3D avatars from videos.
Vid2Avatar, accepted at CVPR 2023 and available on GitHub and arXiv, reconstructs detailed and realistic 3D human models from monocular videos with diverse backgrounds and camera motions. Unlike previous methods, Vid2Avatar doesn’t require ground truth supervision or prior knowledge of human shape and pose, making it invaluable for various applications.
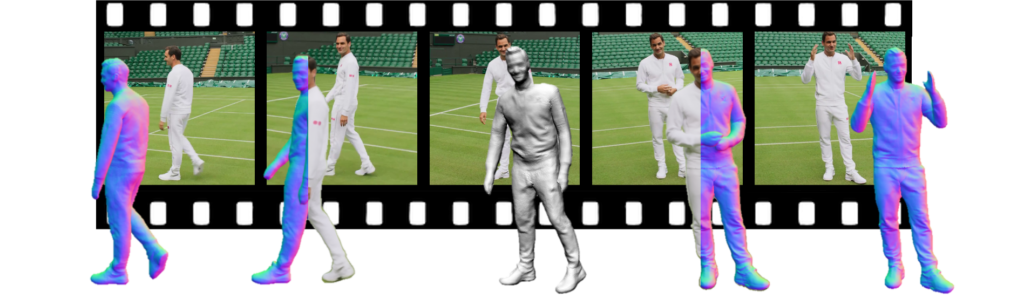
Separating Human and Background in 3D Space
Innovative Approach: Vid2Avatar’s Key Idea
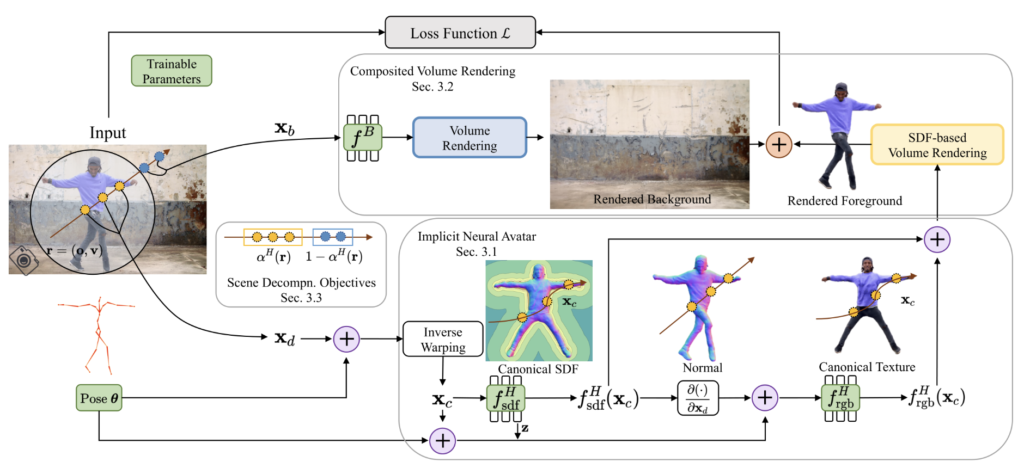
To reconstruct detailed geometry and appearance of implicit neural avatars from monocular videos in the wild, Vid2Avatar tackles the tasks of scene decomposition and surface reconstruction directly in 3D. This sets it apart from prior works that rely on off-the-shelf 2D segmentation tools or manually labeled masks. Vid2Avatar achieves this by modeling both the human and background in the scene implicitly, using two separate neural fields that are learned jointly from images to composite the entire scene. To enhance the delineation of surfaces and alleviate the ambiguity of in-contact body and scene parts, Vid2Avatar introduces novel objectives that leverage the dynamically updated human shape in canonical space to regulate the ray opacity.
Impressive Results and Comparisons
Evaluation and Outperforming Existing Methods
To validate the effectiveness of Vid2Avatar, the authors conducted extensive evaluations using prominent datasets such as Human3.6M, SURREAL, and YouTube videos. Comparing Vid2Avatar with existing methods such as Neural Body, Neural Volumes, and ROMP, the results were astounding. Vid2Avatar outperformed its counterparts by generating more accurate and realistic 3D human models, exhibiting fewer artifacts and occlusions. Furthermore, the authors presented qualitative results from custom videos, emphasizing the diversity and robustness of Vid2Avatar’s capabilities.
Results
3D avatar creation from videos
Accessible Implementation and Application
Making Vid2Avatar Accessible to All
What sets Vid2Avatar apart is its accessibility. The code, installation instructions, and execution guidelines are readily available on GitHub. This user-friendly approach empowers anyone interested in 3D avatar reconstruction to easily experiment with Vid2Avatar and witness its remarkable outputs firsthand.
Conclusion
Vid2Avatar represents a significant leap forward in the field of 3D human reconstruction from videos. Its self-supervised scene decomposition approach, combined with the coarse-to-fine optimization technique, sets it apart from existing methods. If you’re intrigued by Vid2Avatar, we highly encourage you to explore their paper, video, and project page. Prepare to embark on an exciting journey of 3D avatar reconstruction from videos with Vid2Avatar, and unlock its vast potential for animation, gaming, virtual reality, and beyond.